AI is changing how online stores help their customers. It’s making customer service faster, more personal, and available all the time. Let’s look at how AI is improving e-commerce customer service and the top tools that are leading this change. These tools are setting new standards for how businesses talk to customers and make online shopping better.
Key AI Tools for E-commerce Customer Service
- AI-Powered Chatbots
- Natural Language Processing (NLP) Systems
- Predictive Analytics Tools
- Automated Email Response Systems
- Visual Search AI
- Voice-Activated Assistants
- Sentiment Analysis Tools
- Personalization Engines
- AI-Driven Recommendation Systems
- Automated Inventory Management
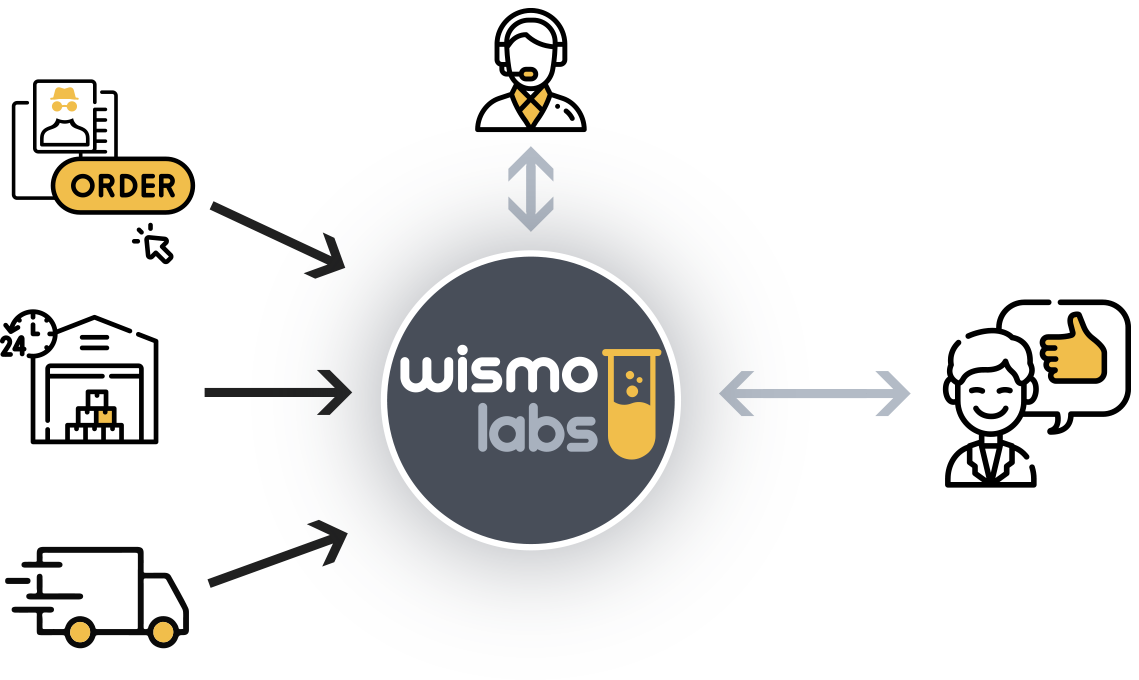
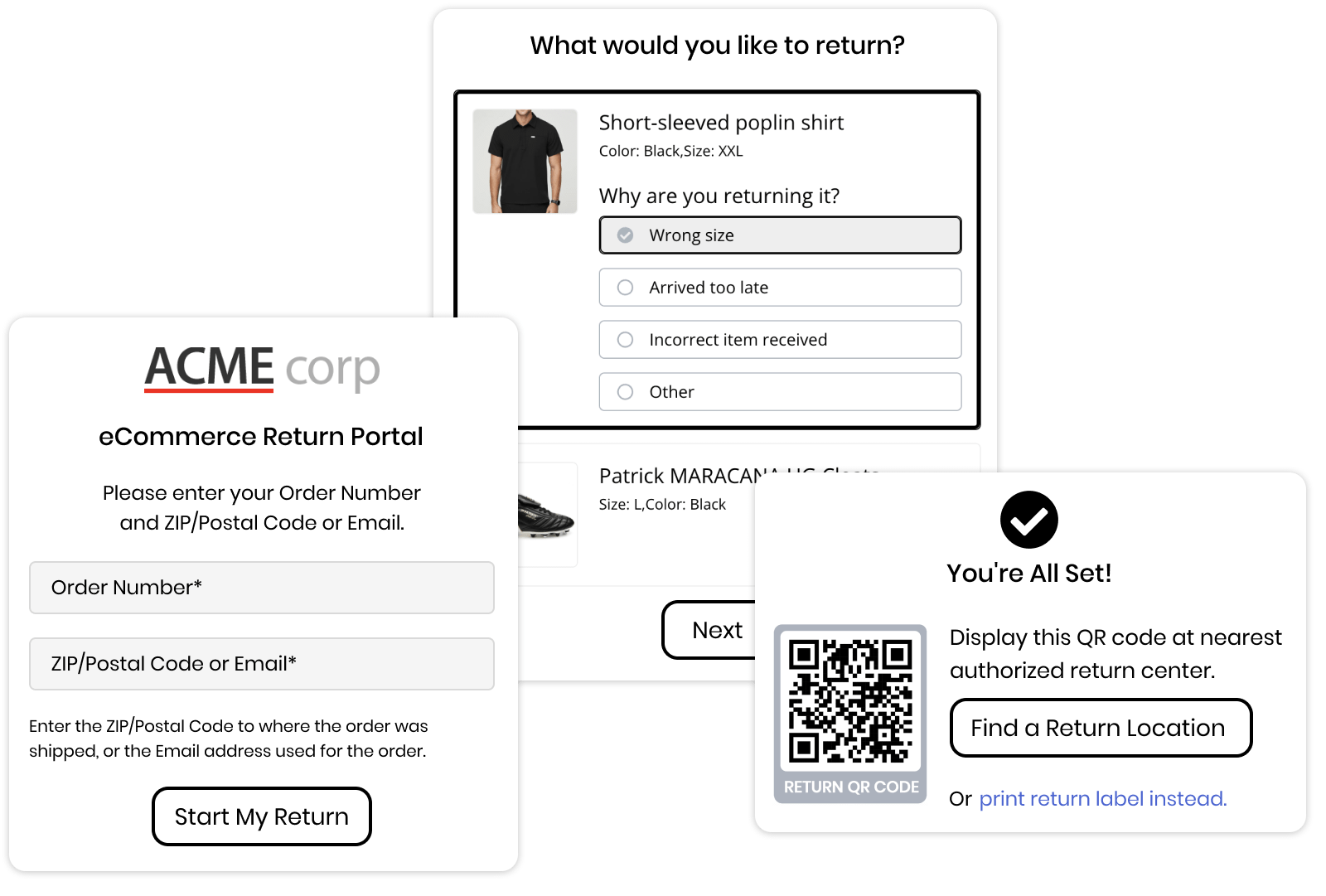
The AI Revolution in E-commerce Customer Service
AI is changing how online stores talk to their customers. It uses smart technology to make customer support better, more personal, and quicker. This change is big and is changing how businesses help their customers. Here’s how AI is making a difference:
- Always available to help customers, no matter what time it is
- Creates shopping experiences just for you, based on what you like
- Answers simple questions automatically, so human helpers can focus on harder problems
- Solves problems faster, making customers happier
- Guesses what customers might need before they ask
- Helps businesses grow without needing to hire lots more people
These improvements are not just making customers happier but also helping businesses work better. With AI handling easy tasks, human helpers can work on trickier problems that need a personal touch. This mix of AI and human help is creating a new, better way of helping customers. Also, the information AI tools provide helps businesses keep improving their products and how they talk to customers.
Top AI Tools Transforming E-commerce Customer Service
Let’s look at some of the best AI tools that are making customer service in online stores much better. These tools use the latest AI technology to help with customer support, each solving specific problems in online shopping:
1. AI-Powered Chatbots
Chatbots are like digital helpers that many online stores use to talk to customers. These AI helpers can answer lots of different questions, from telling you about products to tracking orders. The newest chatbots are really smart and keep getting better at helping customers. Here’s why chatbots are so great:
- They can answer questions right away, any time of day or night
- They can talk to many customers at once, which helps a lot when it’s busy
- They always give the same correct information, which humans might sometimes get wrong
- They learn from every conversation, so they get better over time
- If a question is too hard, they can smoothly pass it to a human helper
- They collect useful information about what customers are asking, which helps businesses improve
WISMOlabs’ tracking solutions work well with AI chatbots, letting customers check where their packages are without needing to talk to a person. This shows how AI can make specific parts of online shopping better, giving customers quick answers about important things like their orders. By answering these common questions automatically, businesses can help customers faster and make them happier, while also letting human helpers focus on harder questions. This not only makes customers happier but also helps the business work more efficiently.
2. Natural Language Processing (NLP) for Better Communication
Natural Language Processing is technology that helps AI understand and respond to how people talk naturally. In online store customer service, NLP is making it much easier for businesses to talk with their customers, breaking down barriers between how humans speak and what machines can understand. This technology is really important for making conversations between customers and AI systems feel more natural. Here’s how NLP is helping:
- Understands what customers mean, not just the exact words they use
- Gives answers that make sense for the conversation, making it feel more like talking to a person
- Can understand many languages, helping businesses talk to customers around the world
- Figures out how customers are feeling to give better answers
- Helps with voice assistants, so customers can ask for help by speaking
- Makes it easier for customers to find products or information they’re looking for
WISMOlabs’ NLP for shipping support shows how this technology can make answering questions about shipping and delivery much easier. By understanding complex questions and giving accurate information, NLP systems are making customer support work much better. This use of NLP shows how it can handle specific, often technical, parts of online shopping support, making sure customers get exact and helpful information without always needing to talk to a person. NLP’s ability to understand questions asked in normal language means customers can ask in their own words, making getting help easier and more user-friendly.
3. AI-Driven Personalization
Making each customer’s experience special is key to great online shopping. AI tools are taking this to a new level by looking at lots of customer information to create experiences just for each person. This kind of personalization used to be impossible for big businesses, but AI has made it possible for stores of all sizes. Here’s how AI-driven personalization is making customer service better:
- Suggests products you might like based on what you’ve looked at and bought before
- Changes how it talks to you based on your history and what you like
- Guesses what you might need and offers help before you ask
- Sends you ads about things you’re actually interested in
- Makes the website look different for each person
- Gives you special discounts on things you might want to buy
WISMOlabs’ personalized marketing tool is a great example of how AI can create special experiences for customers after they buy something. By looking at what customers have bought before, businesses can suggest other things they might like, making customers happier and selling more. This tool shows how powerful AI is in not just answering customer needs but in guessing what they might want next, creating chances for both the customer and the business to get more value. Being able to make marketing this personal means customers see offers and suggestions that really match what they’re interested in, which makes marketing work better and improves the overall shopping experience.
4. AI-Powered Analytics for Customer Insights
AI analytics tools are giving online businesses new ways to understand how customers behave and what they like. This information is really important for making customer service better and helping the whole business do well. By looking at huge amounts of information quickly, AI analytics can find patterns that people would never be able to see on their own. Here’s how AI analytics are making a difference:
- Spots trends in what customers are doing, helping businesses guess what customers will want next
- Helps predict what customers might need in the future, so businesses can get ready
- Helps make smart decisions about everything from what to keep in stock to how to advertise
- Groups customers better so each group gets the right kind of help and offers
- Looks at what customers are saying about the business everywhere, not just on the website
- Finds problems in how customers shop before they become big issues
WISMOlabs’ analytics dashboard for online stores shows how businesses can use AI to learn important things from how customers interact and how shipping is going. This information can be used to make customer support better, improve products, and make the whole shopping experience nicer. By showing important numbers and trends clearly, these analytics tools help businesses make smart decisions quickly. This way of using data to improve customer service and how the business works can lead to big improvements in how well things run, how happy customers are, and how much the business grows.
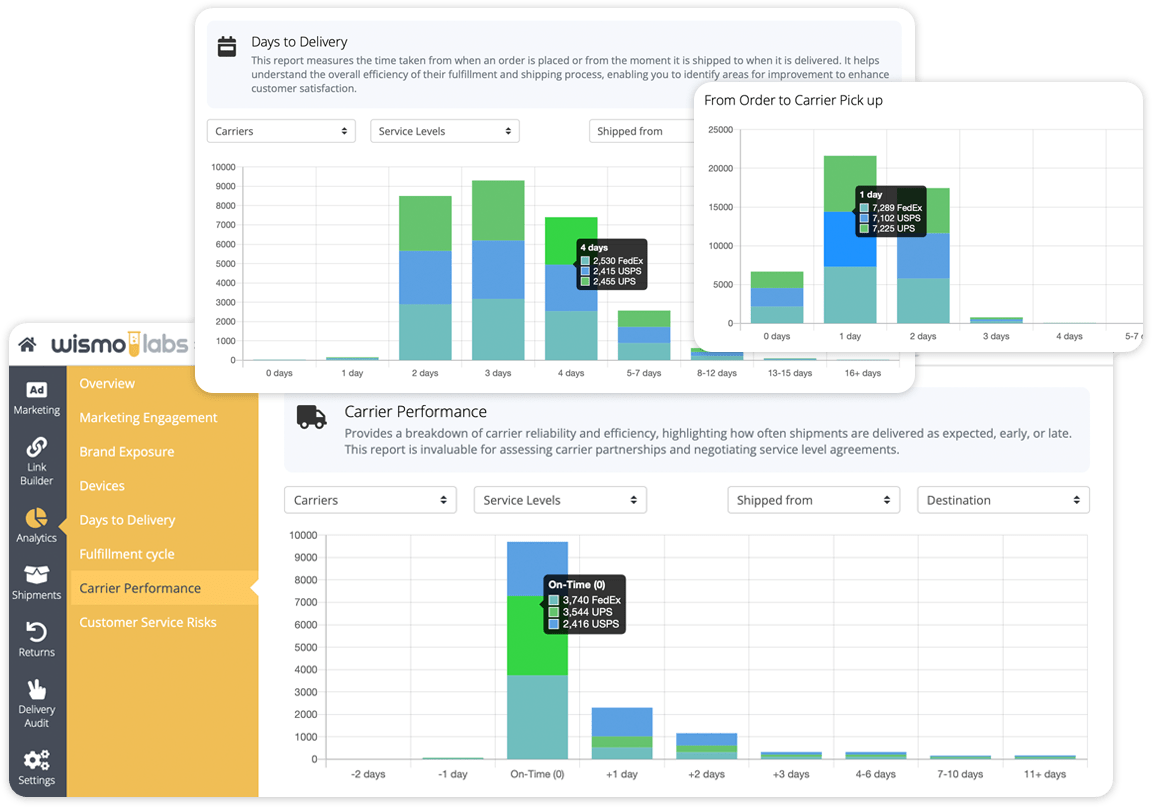
5. AI-Enabled Order and Shipment Tracking
One of the most common things customers ask about in online shopping is where their order is and when it will arrive. AI tools are making this part of customer support much better by giving real-time, accurate information. Adding AI to order and shipment tracking systems has really helped reduce customer worry and the number of questions about order status. Here’s how AI is making order and shipment tracking better:
- Gives real-time updates on tracking, so customers always know the latest about their orders
- Sends messages about shipment status automatically, keeping customers informed without them having to check
- Reduces “Where Is My Order” questions, freeing up customer service to help with harder problems
- Works with many different shipping companies to make tracking easy, no matter who’s delivering the package
- Predicts delivery dates more accurately, using AI to think about all the things that can affect shipping times
- Lets customers easily check tracking information themselves, which means fewer calls to customer service
WISMOlabs’ branded tracking solutions show how AI can be used to create a smooth, branded tracking experience for customers. By giving accurate, up-to-the-minute information, these tools really help reduce customer worry and questions about where orders are. Being able to offer a branded tracking experience also helps businesses keep their brand looking consistent throughout the customer’s journey, making customers more loyal and trusting. Also, by using AI that can predict things, these tracking solutions can tell customers more accurately when their packages will arrive, making the whole shopping experience even better.
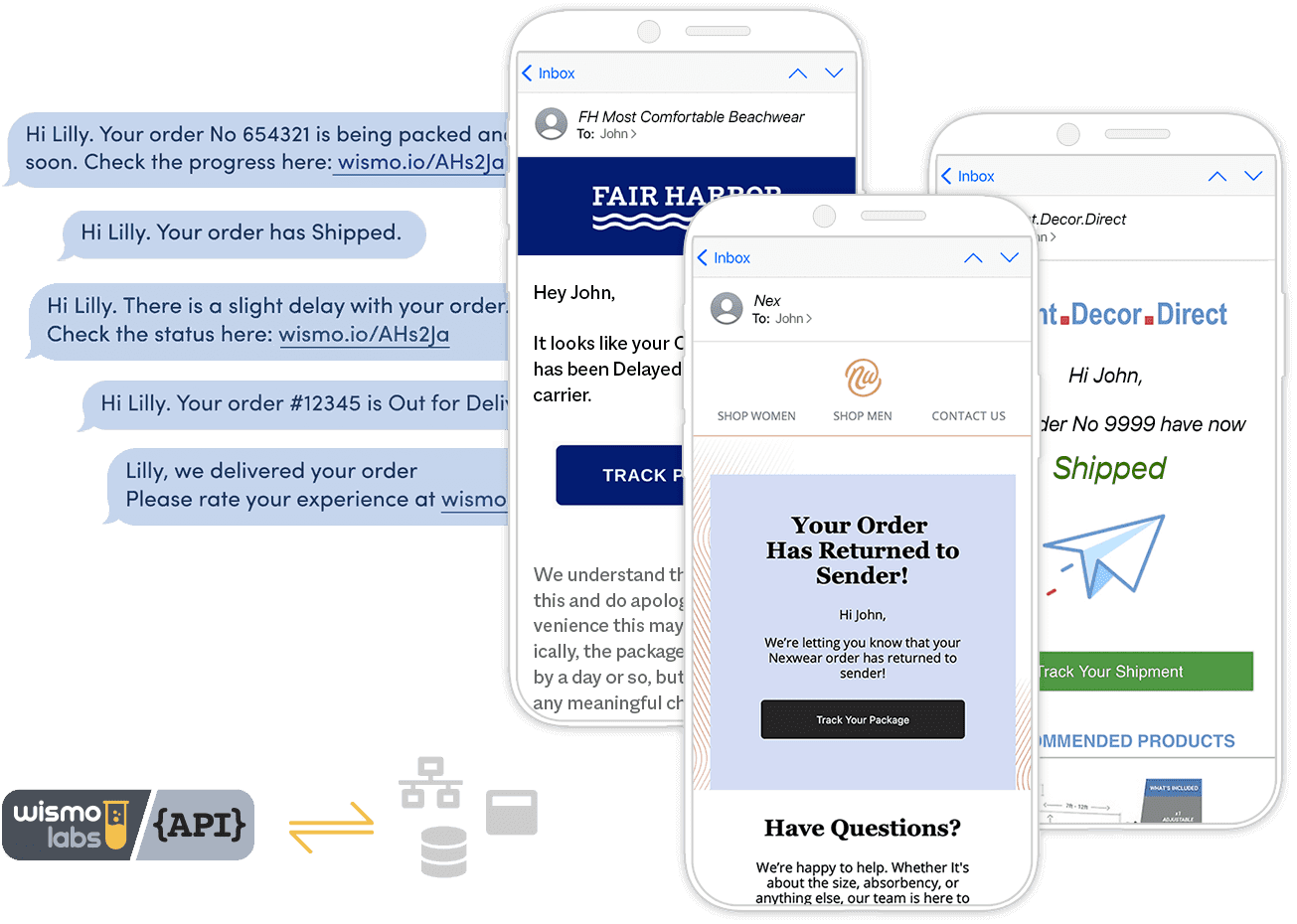
6. AI in Returns Management
Managing returns is a really important part of online store customer service that can really affect how happy customers are and how well the business does. AI tools are making the returns process smoother, making it easier for both customers and businesses. By automating and improving different parts of returns management, AI is helping businesses turn what could be a bad experience into a chance to make customers loyal. Here’s how AI is changing returns management:
- Makes the returns process automatic for faster solutions, making it quicker and easier for customers
- Uses smart analysis to stop returns before they happen by finding patterns in why things are returned
- Offers personalized return options based on what the customer has done before and what kind of product it is
- Makes refund processing faster, ensuring quick and accurate paybacks
- Looks at return data to make product descriptions better and reduce future returns
- Smartly routes returned items to the best place, saving money and managing inventory better
WISMOlabs’ e-commerce returns solution shows how AI can be used to create a smooth returns experience. By automating different parts of the returns process and giving clear, real-time information to customers, businesses can turn what could be a negative experience into a chance to make customers loyal. This AI-driven approach not only makes the customer experience better but also helps businesses work more efficiently, spend less on returns, and learn valuable things about how well products are doing and what customers prefer. Being able to offer an easy, hassle-free returns process can really increase customer trust and encourage them to buy again, even if they weren’t happy with their purchase at first.
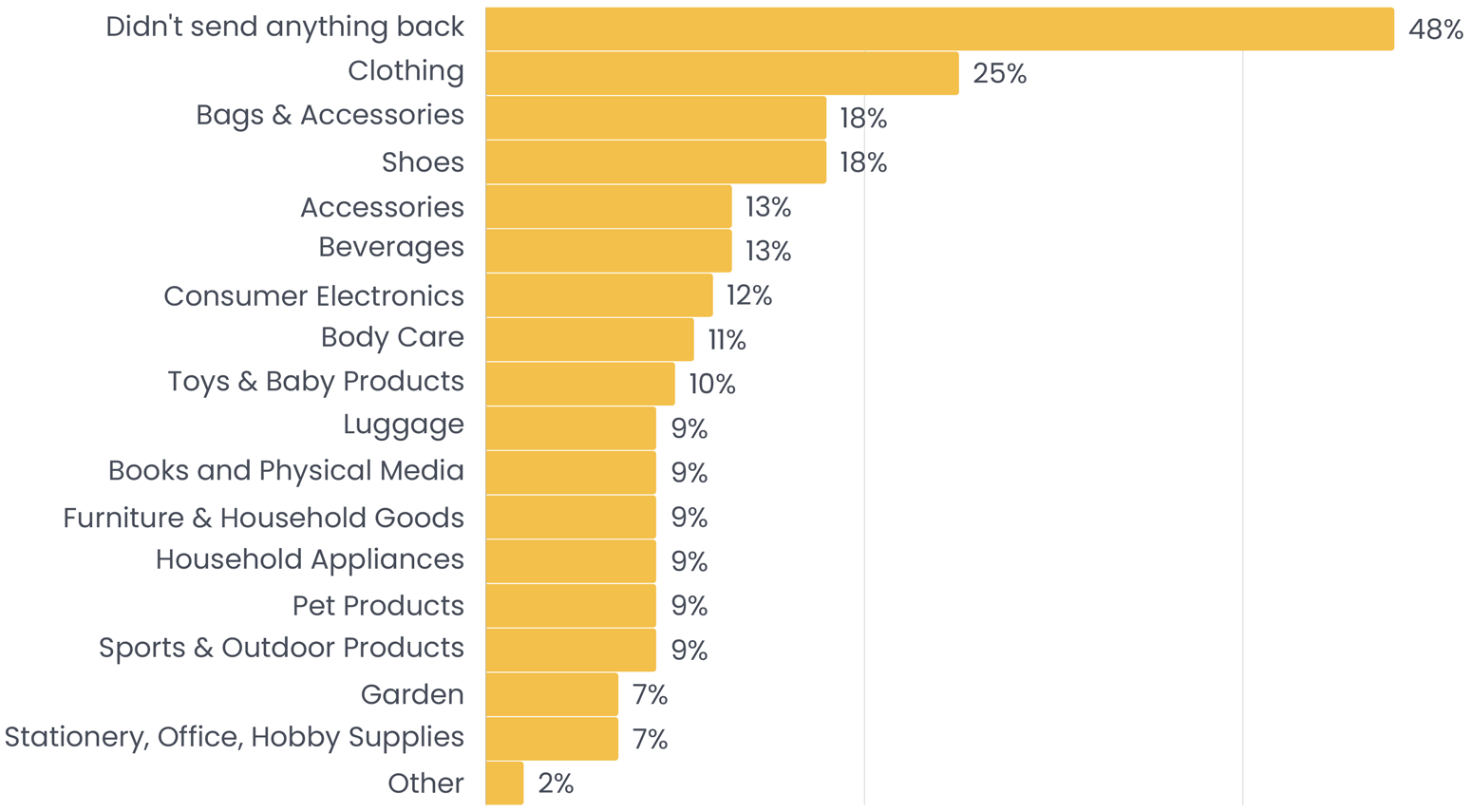
Using AI Tools: Best Ways for Online Stores
While AI tools offer great potential for improving online store customer service, using them successfully requires careful planning and execution. Adding AI to existing business processes should be strategic and match overall business goals. Here are some best ways to use AI tools in your online store:
- Look at your specific business needs and customer problems to see where AI can help the most
- Choose AI tools that work well with your existing systems to ensure smooth data flow and efficient operations
- Make data privacy and security a top priority when using AI, following relevant rules and building customer trust
- Give thorough training to your staff on working with AI tools, emphasizing how AI can help rather than replace human roles
- Start with small test programs to try out AI implementations before using them fully, allowing for adjustments and improvements
- Keep watching how AI performs and get feedback from both customers and employees to refine and improve AI-driven processes
- Be open with customers about using AI in customer service interactions, setting clear expectations and boundaries
It’s important to remember that AI tools are meant to make human abilities better, not replace them completely. The most successful uses of AI in customer service find the right balance between automated efficiency and human empathy. This mixed approach ensures that customers get quick, accurate responses for routine questions while still having access to human support for more complex or sensitive issues. Regularly checking and improving AI performance is also essential to ensure that these tools continue to meet changing customer needs and business goals. By following these best practices, online stores can use AI to significantly improve their customer service operations while keeping the personal touch that is often crucial in building lasting customer relationships.
24/7 Availability
AI tools provide round-the-clock customer service, ensuring support at any time.
Faster Responses
AI chatbots offer instant responses, handling routine queries efficiently.
Personalized Interactions
AI analyzes customer data to provide tailored experiences and recommendations.
Natural Language Processing
NLP enables AI to understand and respond to human language naturally.
The Future of AI in Online Store Customer Service
As AI technology keeps getting better, we can expect even more innovative uses in online store customer service. The rapid improvements in AI and machine learning are opening up new possibilities for making customer experiences better and streamlining business operations. Some emerging trends to watch out for include:
Emerging AI Technologies in Customer Service
Advanced Natural Language Processing
Human-indistinguishable conversations with nuanced understanding of context, cultural references, and emotional subtleties.
Predictive Customer Service
AI systems that anticipate customer needs before they’re expressed, identifying potential issues and proactively offering solutions.
Emotional Intelligence
Sophisticated emotion recognition capabilities that detect subtle cues in text, voice, and visual interactions to deliver empathetic, contextually appropriate responses.
Immersive Technology Integration
Seamless blending of AI with AR/VR, IoT, and voice interfaces to create multi-sensory, intuitive shopping experiences across physical and digital realms.
Next-Generation Natural Language Processing
- Contextual Conversation Memory: Advanced AI systems that maintain comprehensive understanding of customer conversation history across multiple sessions and channels, creating truly continuous relationships that eliminate the need for customers to repeat information.
- Cultural Nuance Recognition: Sophisticated language models trained on diverse cultural contexts that understand regional expressions, idioms, humor, and communication styles, enabling genuinely localized customer service regardless of market.
- Multimodal Understanding: Integrated systems that simultaneously process text, voice, images, and video inputs to comprehend complex customer queries that span multiple forms of communication, such as a customer showing a product issue while describing it.
- Sentiment-Adaptive Dialogue: Dynamic conversation engines that continuously adjust tone, pacing, vocabulary, and information density based on real-time analysis of customer sentiment and engagement signals.
- Zero-shot Problem Solving: Next-generation reasoning capabilities that can effectively handle previously unseen customer issues without explicit programming, drawing on broader knowledge and inference abilities to develop creative solutions.
Augmented Reality Integration
- AI-Guided Virtual Try-On: Intelligent systems that not only enable virtual product trials but actively provide personalized styling advice, fit recommendations, and alternative suggestions based on customer preferences and body measurements.
- Contextual Product Visualization: AR experiences that show products in the customer’s actual environment with AI adjustments for lighting conditions, space constraints, and complementary items they already own.
- Remote Expert Assistance: Mixed reality interfaces where AI customer service agents can see what customers see and annotate their real-world environment to provide visual guidance for product assembly, troubleshooting, or optimal placement.
- Interactive Product Education: AI-driven AR experiences that adapt product demonstrations based on the customer’s level of familiarity, learning style, and specific usage scenarios, creating personalized product education.
- Social Shopping Layers: Collaborative AR environments where AI facilitates shared shopping experiences between friends or family members in different locations, including virtual consultations with style advisors or product experts.
Predictive Customer Service Capabilities
- Usage Pattern Analysis: AI systems that monitor product usage data (when available) to identify potential issues before they become problems, triggering proactive support interventions or maintenance recommendations.
- Behavioral Prediction Models: Advanced algorithms that analyze browsing patterns, purchase history, and support interactions to anticipate customer needs and questions, preparing relevant resources before customers even reach out.
- Satisfaction Trajectory Mapping: Continuous monitoring of customer sentiment indicators across interactions to predict potential dissatisfaction early in the relationship, enabling preemptive relationship-saving interventions.
- Problem Clustering Detection: Pattern recognition systems that identify emerging product issues by detecting subtle relationships between seemingly isolated customer inquiries, enabling systemic fixes before problems become widespread.
- Life Event Anticipation: Sophisticated models that recognize signals indicating major life changes (moving, having children, changing jobs) and proactively suggest relevant products or services that align with the customer’s new circumstances.
Hyper-Personalization Frameworks
- Psychological Preference Modeling: AI systems that develop comprehensive models of individual customer preferences, communication styles, decision-making patterns, and value hierarchies to tailor every aspect of the shopping experience.
- Dynamic Content Generation: Automated creation of personalized product descriptions, marketing messages, and support content that emphasizes the specific features and benefits most relevant to each customer’s unique needs and interests.
- Interface Adaptation: Self-modifying user interfaces that adjust layout, information density, visual elements, and navigation patterns based on observed user behavior and preferences without requiring explicit customization.
- Cross-Channel Experience Continuity: Unified personalization engines that maintain consistent customer understanding across websites, mobile apps, voice assistants, in-store experiences, and customer service interactions.
- Transparent Personalization Controls: Intuitive interfaces that give customers visibility into how and why their experience is being personalized, with granular controls to adjust algorithms and data usage according to their comfort level.
Voice Commerce Advancements
- Conversational Shopping Assistants: Voice-first AI shopping agents that engage in natural, multi-turn conversations about products, helping customers narrow options through dialogue rather than traditional search and filter paradigms.
- Voice Biometric Authentication: Secure, friction-free payment and account management through advanced voice recognition systems that identify customers based on unique vocal characteristics while detecting potential voice spoofing attempts.
- Multimodal Voice Shopping: Integrated systems that combine voice interaction with visual displays, allowing customers to use natural language for broad exploration while seeing visual results and using touch for precision selections.
- Ambient Commerce Interfaces: Always-available shopping assistants embedded in smart home devices that learn household consumption patterns and enable contextual, need-based purchasing through casual conversation.
- Voice-Driven Product Discovery: Advanced search algorithms optimized for spoken queries that understand nuanced product requirements expressed conversationally, even when customers don’t know exact product terminology.
Emotional Intelligence Systems
- Multimodal Emotion Recognition: Sophisticated systems that detect emotional states through combined analysis of text sentiment, voice tone, facial expressions, and even physiological indicators from wearable devices when available.
- Adaptive Emotional Response: AI service agents with dynamic emotional intelligence that adjust communication style, tone, and content based on detected customer emotions, mimicking the empathetic responses of skilled human agents.
- Emotional Journey Mapping: Analytics platforms that track emotional states throughout the customer journey, identifying emotional pain points and delight moments to guide experience optimization.
- Frustration Prediction and Mitigation: Proactive systems that recognize early indicators of customer frustration and automatically adjust the experience to prevent negative emotions, such as simplifying processes or offering additional assistance.
- Emotional Context Awareness: Advanced AI that considers broader emotional contexts beyond the immediate interaction, such as seasonal stresses, regional events, or personal milestones that might influence a customer’s emotional state.
IoT and Connected Device Integration
- Product Performance Monitoring: Smart products that continuously stream usage and performance data to AI systems, enabling proactive customer service interventions when potential issues are detected before customers notice problems.
- Consumption Pattern Analysis: Connected household devices that track consumption of consumable products and automatically initiate reordering or suggest subscription services based on usage patterns.
- Contextual Service Delivery: AI systems that leverage data from multiple IoT devices to understand the customer’s current context and deliver appropriately timed and relevant service interactions.
- Predictive Maintenance Scheduling: Intelligent algorithms that analyze product sensor data to predict optimal timing for maintenance or replacement, initiating service recommendations that prevent disruptive failures.
- Environmental Adaptation: Smart retail systems that adjust product recommendations and information based on real-time environmental data from connected sensors, such as suggesting weather-appropriate items based on local conditions.
Emerging Technology Convergence
- Blockchain-Verified Customer History: Distributed ledger systems that give customers ownership and control of their service history across multiple retailers, allowing them to selectively share verified purchase and preference data for improved service.
- Edge Computing Service Delivery: Ultra-responsive AI customer service capabilities powered by edge computing that process customer interactions locally, enabling instantaneous responses even with complex queries or limited connectivity.
- Quantum-Enhanced Personalization: Future application of quantum computing to process vastly more complex customer behavior models, identifying subtle patterns in preferences and needs that current systems cannot detect.
- Decentralized Autonomous Service Organizations: Blockchain-based systems where customer service policies, responses, and improvements are governed by transparent smart contracts with customer participation in service evolution.
- Metaverse Customer Experience: Immersive virtual environments where customers can interact with products, service representatives, and other customers in shared spaces that transcend the limitations of current digital interfaces.
These advancements promise to further streamline customer service operations while providing increasingly personalized and satisfying customer experiences. Combining AI with other new technologies like blockchain and edge computing may also lead to new innovations in areas such as secure transactions and real-time data processing. As online stores prepare for this AI-driven future, it’s important to stay informed about the latest developments and continuously evaluate how new AI tools can be used to improve customer service and drive business growth. The businesses that can successfully adapt to and implement these emerging AI technologies will be well-positioned to lead in the competitive online shopping landscape, offering unparalleled customer experiences and operational efficiency.
Conclusion: The AI-Powered Future of E-commerce Customer Service
The integration of AI tools in e-commerce customer service represents a fundamental shift in how online businesses connect with their customers. This technological evolution has transformed customer support from a reactive necessity into a proactive strategic advantage. From sophisticated chatbots and NLP systems to predictive analytics and personalized marketing, AI is enabling businesses to deliver customer experiences that are faster, more intuitive, and tailored to individual preferences at a scale previously unimaginable.
Transformative Impact of AI on Customer Experience
- Shifts customer service from reactive problem-solving to proactive engagement
- Elevates personalization from generic segmentation to truly individual experiences
- Transforms the post-purchase journey into a strategic touchpoint for building loyalty
- Creates a seamless omnichannel experience across all customer interactions
- Enables predictive support that addresses issues before customers experience them
- Balances automation efficiency with the essential human elements of empathy and connection
Tools like WISMOlabs’ tracking solutions and personalized marketing platforms demonstrate how AI can address specific e-commerce challenges with remarkable precision. These practical applications showcase the versatility of AI in enhancing every aspect of customer interaction, from answering routine queries to predicting future needs and preferences.
The Human-AI Partnership
The most successful implementations of AI in customer service recognize that technology and human touch are complementary forces rather than competing alternatives. While AI excels at speed, consistency, and data processing, human agents bring emotional intelligence, creative problem-solving, and genuine connection to complex interactions. This synergy creates a customer service ecosystem where each component operates at its highest potential.
Strategic Implementation
Successful adoption of AI customer service tools requires thoughtful integration with existing systems, clear alignment with business objectives, and ongoing refinement based on performance metrics and customer feedback. Organizations that approach AI as a strategic investment rather than a quick technological fix will realize the greatest benefits in customer satisfaction and operational efficiency.
As we look toward the future, the evolution of AI in e-commerce customer service will continue to accelerate. Emerging technologies like emotional intelligence systems, voice commerce, and AR integration promise to create even more intuitive and immersive customer experiences. The businesses that thrive in this new landscape will be those that embrace these technologies while maintaining a clear focus on customer needs and preferences.
The integration of AI in e-commerce customer service is not merely a technological upgrade—it represents a fundamental reimagination of how businesses connect with their customers. By embracing these powerful tools and balancing automation with authentic human connection, e-commerce businesses can create customer experiences that are not just satisfactory but truly exceptional. In the competitive online marketplace, this commitment to service excellence through thoughtful AI implementation may well be the defining factor between businesses that merely survive and those that truly thrive.
Having the right tools to analyze shipment data is crucial for success in logistics and e-commerce. As we look to 2025, efficient data analysis will be key to optimizing shipping operations. New technologies are emerging and existing ones are becoming more advanced, allowing businesses to gain deeper insights, make smarter decisions, and significantly improve their efficiency. Let’s explore the top 10 tools that will transform shipment data analysis in the coming years, looking at their features, benefits, and potential impact on the logistics industry.
Key Takeaways |
---|
• AI and machine learning are driving predictive analytics in shipping • Real-time tracking and visibility solutions are becoming essential • Blockchain technology is enhancing supply chain transparency • IoT is revolutionizing shipment monitoring and condition tracking • Advanced returns management systems are improving customer satisfaction • Sustainability-focused analytics are gaining importance in shipping |
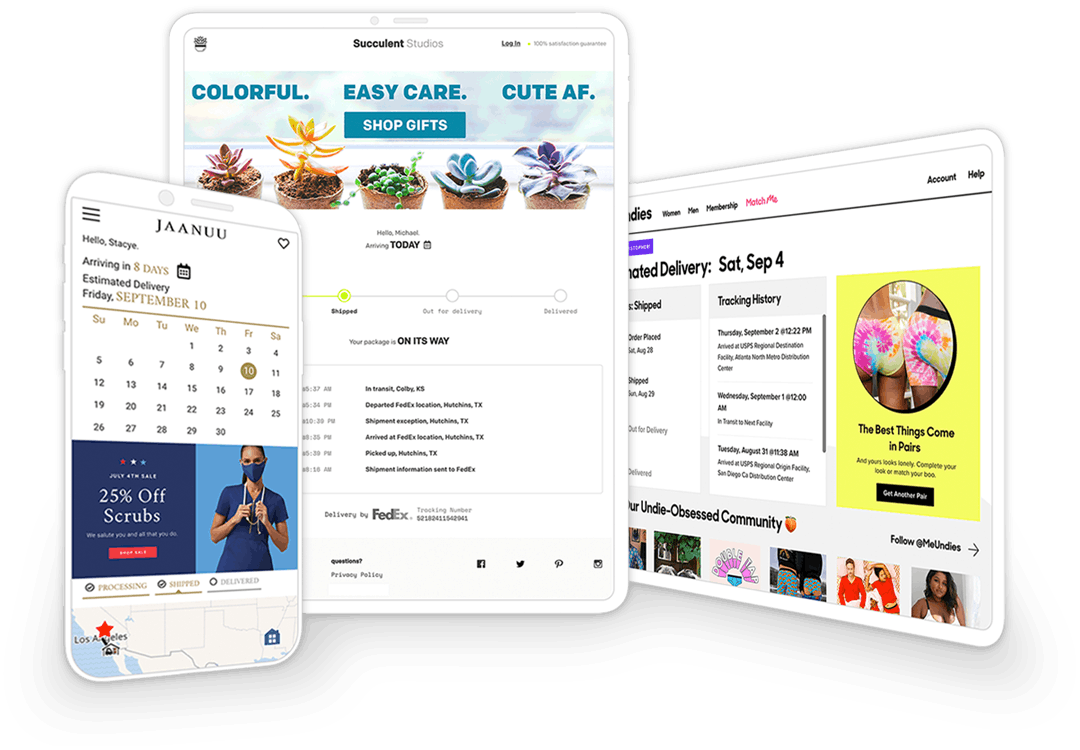
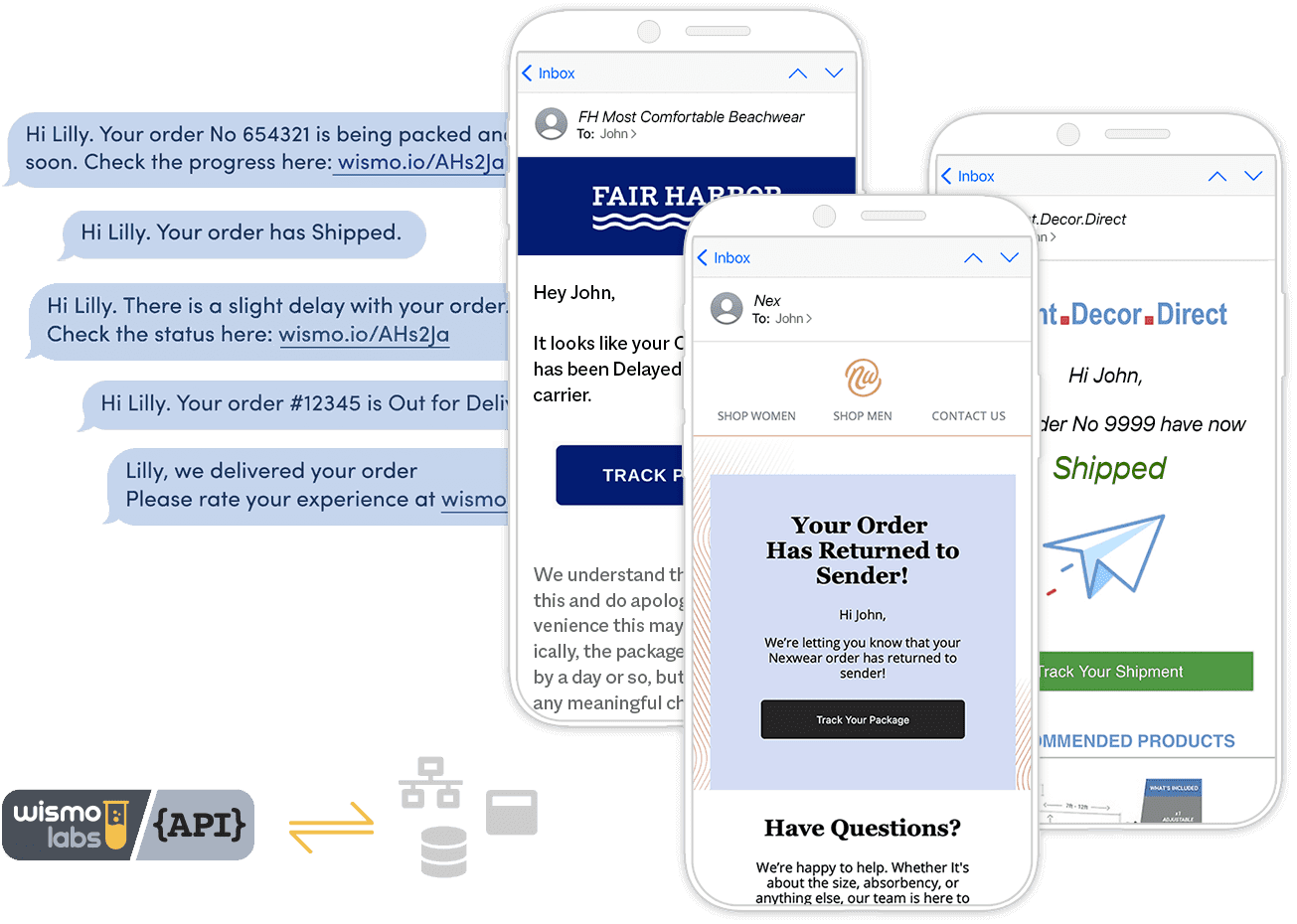
WISMOlabs: The All-in-One Shipment Data Analysis Solution
WISMOlabs stands out as a comprehensive platform for analyzing shipment data, giving businesses powerful insights to improve their logistics operations. This innovative tool addresses the complex needs of modern e-commerce and logistics companies. WISMOlabs takes a holistic approach to shipment data analysis, looking at every part of the shipping process from order placement to final delivery and returns. This tool offers:
- Automated eCommerce returns management, which simplifies handling returns, cutting operational costs and improving customer satisfaction
- User-friendly, branded self-service portal that enhances the customer experience for tracking shipments and managing orders
- Seamless integration with major e-commerce platforms, allowing easy incorporation into existing technology setups
- Real-time tracking and analytics providing up-to-the-minute insights into shipment status, enabling proactive problem-solving
- Advanced data visualization tools that make complex shipment data easy to understand, helping with quicker decision-making
With WISMOlabs, businesses can optimize their eCommerce returns process and gain valuable insights into their shipping performance. The platform’s comprehensive approach to data analysis helps companies spot trends, identify inefficiencies, and implement data-driven strategies to improve their overall logistics operations. By using WISMOlabs’ advanced analytics capabilities, businesses can boost their operational efficiency and significantly improve customer satisfaction through more accurate and timely shipment information.
AI-Powered Predictive Analytics Platform
As we move towards 2025, artificial intelligence will play a big role in shipment data analysis. An AI-powered predictive analytics platform represents a major step forward in logistics technology, offering new capabilities in forecasting, optimization, and decision-making. These advanced systems use machine learning algorithms and lots of historical and real-time data to provide actionable insights and predictions. An AI-powered predictive analytics platform offers:
- Advanced demand forecasting that uses complex algorithms to analyze historical data, market trends, and external factors to predict future shipping volumes accurately
- Route optimization using historical data and real-time conditions, considering things like traffic patterns, weather, and delivery time windows to suggest the most efficient routes
- Predictive maintenance for shipping equipment, which analyzes performance data to predict potential failures before they happen, reducing downtime and maintenance costs
- Dynamic pricing models that adjust shipping rates in real-time based on demand, capacity, and market conditions, maximizing profitability while staying competitive
- Risk assessment and mitigation strategies that identify potential supply chain disruptions and suggest proactive measures to minimize their impact
These tools will help businesses enhance their DHL tracking capabilities and make data-driven decisions to improve overall shipping efficiency. By using AI-powered predictive analytics, companies can anticipate challenges, optimize resources, and stay ahead of market trends, ultimately leading to improved customer satisfaction and increased profitability. The integration of AI into shipment data analysis marks a new era in logistics management, where decisions are increasingly driven by sophisticated predictive models rather than reactive measures.
Real-Time Tracking and Visibility Solution
In 2025, real-time tracking will be more important than ever. As customers expect faster and more transparent shipping, businesses must adopt cutting-edge visibility solutions to stay competitive. These advanced systems go beyond simple package tracking, offering a comprehensive view of the entire supply chain in real-time. A cutting-edge visibility solution provides:
Evolution of Supply Chain Visibility
Past: Milestone Updates
Basic location scans at limited checkpoints with delayed updates and minimal predictive capabilities.
Present: Real-Time Tracking
Continuous GPS-based location data with IoT sensor integration and AI-powered delivery predictions.
Integrated Supply Networks
End-to-end visibility across production, warehousing, transportation, and last-mile delivery with proactive exception management.
Future: Autonomous Optimization
Self-adapting logistics networks with predictive rerouting, automated exception handling, and customer-specific delivery optimization.
Advanced Location Tracking Technologies
- Multi-Signal Triangulation: Sophisticated systems that combine GPS, cellular networks, Wi-Fi positioning, and Bluetooth beacons to maintain continuous visibility even in traditionally challenging environments like dense urban areas or indoor facilities.
- IoT Sensor Integration: Network of interconnected sensors that monitor not only location but also environmental conditions such as temperature, humidity, light exposure, and shock events, providing comprehensive shipment condition visibility.
- Mesh Network Connectivity: Resilient tracking infrastructure using mesh networking protocols that allow packages to communicate with nearby devices when traditional network connectivity is limited, creating redundant data transmission paths.
- Ultra-precise Geolocation: Advanced positioning technologies that provide meter-level accuracy even in challenging environments, enabling precise indoor warehouse tracking and last-foot delivery location data.
- Battery-efficient Tracking: Long-life tracking devices utilizing energy harvesting and ultra-low power consumption technologies that enable continuous real-time tracking throughout extended supply chain journeys without requiring recharging.
Predictive Delivery Estimation Capabilities
- Multi-factor ETA Modeling: Machine learning algorithms that incorporate real-time traffic data, weather conditions, carrier performance patterns, facility throughput rates, and dozens of other variables to generate highly accurate delivery window predictions.
- Dynamic Recalculation Engine: Continuous ETA refinement systems that automatically adjust delivery predictions as conditions change, providing increasingly precise time windows as the shipment nears its destination.
- Carrier-specific Performance Adjustment: Advanced analytics that account for historical performance patterns of specific carriers, routes, and even individual drivers to fine-tune standard industry delivery estimates with company-specific accuracy factors.
- Delivery Density Impact Analysis: Sophisticated models that account for how delivery density in specific areas affects last-mile timing, adjusting predictions based on the current volume of deliveries scheduled for neighboring locations.
- Customer Availability Matching: AI systems that correlate delivery timing with customer availability patterns to suggest optimal delivery windows that maximize successful first-attempt deliveries while maintaining logistical efficiency.
Proactive Alert and Exception Management
- Predictive Delay Detection: Machine learning systems that identify potential delivery issues before they occur by analyzing patterns in carrier movements, weather forecasts, and traffic conditions, enabling preemptive intervention.
- Intelligent Alert Prioritization: Sophisticated algorithms that classify shipping exceptions based on customer impact, business priority, and resolution urgency, ensuring that the most critical issues receive immediate attention.
- Automated Resolution Workflows: Pre-programmed intervention pathways that automatically initiate appropriate actions based on the nature of the shipping exception, such as rerouting packages, scheduling alternative delivery options, or proactively communicating with customers.
- Conditional Notification Rules: Customizable alert engines that determine which stakeholders should be notified about specific types of exceptions based on shipment value, customer segment, product type, and other business-relevant factors.
- Resolution Performance Analytics: Comprehensive tracking of how different exception types are resolved, measuring time-to-resolution, resource requirements, and customer satisfaction impact to continuously improve exception management processes.
Inventory Integration Capabilities
- In-Transit Inventory Visibility: Real-time tracking of all inventory currently moving through the supply chain, providing accurate snapshots of available stock including goods in transit between facilities or en route from suppliers.
- Dynamic Safety Stock Calculation: Automated adjustments to safety stock requirements based on current in-transit visibility, transit time reliability, and demand volatility to optimize inventory levels while maintaining service levels.
- Cross-docking Optimization: Intelligent systems that identify opportunities to redirect incoming shipments directly to outbound transportation, bypassing warehouse storage and reducing handling costs while accelerating delivery.
- Virtual Allocation Management: Advanced algorithms that manage the assignment of in-transit inventory to customer orders or production requirements before physical arrival, maximizing inventory utilization and fulfillment speed.
- Supply Chain Event Simulation: Predictive modeling that demonstrates how potential disruptions would impact inventory positions across the network, enabling proactive inventory positioning decisions to mitigate risks.
Analytics Dashboard Capabilities
- Carrier Performance Benchmarking: Comprehensive analytics that compare multiple carriers across various performance dimensions including on-time delivery, exception rates, cost efficiency, and damage frequency, with drill-down capabilities for route-specific analysis.
- Delivery Density Optimization: Heat map visualizations that identify optimal delivery routing and scheduling patterns based on geographical concentration of deliveries, enabling more efficient last-mile operations.
- Predictive Transit Modeling: Machine learning algorithms that analyze historical shipping data to identify patterns and predict future transit times across different lanes, helping optimize inventory positioning and customer promises.
- Cost Leakage Identification: Advanced algorithms that detect instances where actual shipping costs exceed expected costs due to service upgrades, address corrections, or other avoidable surcharges, highlighting opportunities for process improvement.
- Carbon Footprint Tracking: Sustainability metrics that calculate and visualize the environmental impact of different shipping options and routes, supporting more environmentally responsible logistics decision-making.
Customer Experience Enhancements
- Interactive Delivery Control: Customer-facing interfaces that enable recipients to modify delivery timing, location, or special instructions in real-time as packages are en route, providing unprecedented control over the delivery experience.
- Augmented Reality Visualization: Mobile applications that use AR technology to show customers exactly where their package is and its expected path to delivery, creating an engaging and transparent tracking experience.
- Delivery Confidence Indicators: Visual representations of delivery prediction confidence based on historical accuracy of similar shipments, giving customers realistic expectations about potential delivery timing variations.
- Seamless Delivery Handoffs: Integration with smart home devices, secure access systems, and pickup location networks to facilitate convenient and secure delivery regardless of recipient availability.
- Personalized Communication Cadence: AI-driven communication systems that learn individual customer preferences for tracking update frequency and detail level, delivering the right information through preferred channels at the optimal time.
Implementation Best Practices
- Phased Deployment Strategy: Structured implementation approach that begins with core visibility functionality for high-value shipments before expanding to cover all shipments and additional advanced features, ensuring sustainable adoption and value realization.
- Multi-stakeholder Integration: Comprehensive engagement with all departments including sales, customer service, logistics, and finance during implementation to ensure the visibility solution addresses diverse business needs and use cases.
- Data Governance Framework: Robust data management protocols that maintain data quality, security, and privacy throughout the tracking ecosystem, with clear policies for data retention, access controls, and compliance requirements.
- Carrier Collaboration Program: Structured approach to engaging shipping partners in the visibility initiative, including technical integration standards, performance metrics, and mutual benefit frameworks to encourage active participation.
- Continuous Improvement Cycle: Systematic process for gathering user feedback, measuring business outcomes, and implementing regular enhancements to the visibility platform based on evolving business needs and technological capabilities.
This tool will be essential for businesses looking to improve their FedEx tracking processes and provide customers with up-to-the-minute shipment information. Real-time tracking and visibility solutions not only enhance the customer experience by providing transparent and accurate shipping information but also enable businesses to optimize their logistics operations, reduce costs, and improve overall efficiency. As supply chains become more complex and global, the ability to maintain real-time visibility across all touchpoints will be a key differentiator for successful businesses in the logistics and e-commerce sectors.
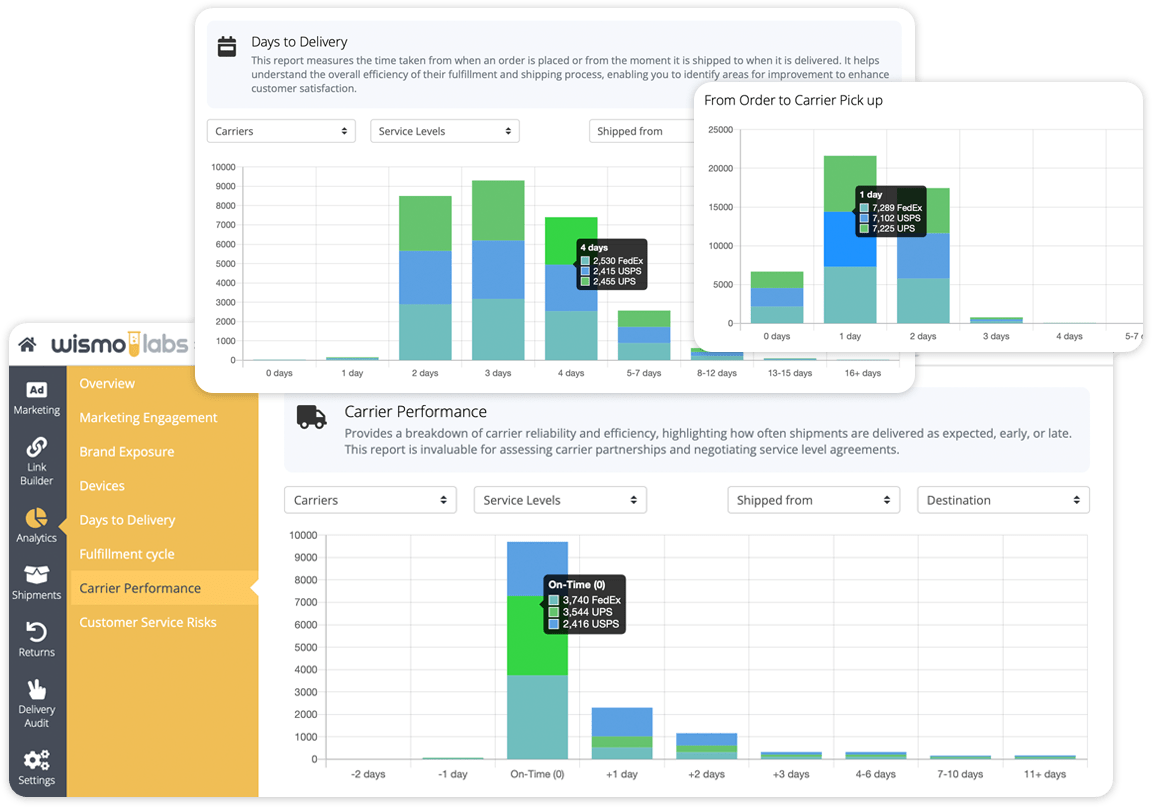
Carrier Performance Analytics Tool
Understanding how different carriers perform is essential for optimizing shipping strategies. In a competitive logistics landscape, businesses need to make informed decisions about which carriers to use for different types of shipments and routes. A robust carrier performance analytics tool offers comprehensive insights that go beyond basic metrics, enabling businesses to make data-driven decisions that optimize their shipping operations. This advanced tool offers:
- Comparative analysis of carrier performance, allowing businesses to evaluate multiple carriers based on various metrics such as on-time delivery rates, handling times, and customer satisfaction scores
- Cost-efficiency metrics that consider not just shipping rates but also hidden costs such as insurance, fuel surcharges, and handling fees to provide a true picture of carrier costs
- On-time delivery rate tracking across different regions, shipment types, and time periods, enabling businesses to identify trends and make informed carrier selections
- Detailed analysis of exception events, such as delays, damages, and lost shipments, helping businesses understand the root causes of issues and work with carriers to improve performance
- Customizable reporting tools that allow businesses to create tailored reports focusing on the metrics that matter most to their specific operations
This tool will help businesses optimize their UPS tracking and overall carrier performance. By using detailed carrier performance analytics, companies can negotiate better rates, improve service level agreements, and ultimately enhance their shipping operations. The insights provided by these tools enable businesses to make strategic decisions about carrier selection, route optimization, and resource allocation, leading to improved efficiency, reduced costs, and enhanced customer satisfaction. As shipping becomes an increasingly critical component of business success, particularly in the e-commerce sector, the ability to accurately assess and optimize carrier performance will be a key competitive advantage.
Blockchain-Enabled Supply Chain Transparency Platform
By 2025, blockchain technology will be widely used in shipment data analysis, changing how businesses manage and track their supply chains. Blockchain’s key features of immutability, transparency, and decentralization make it ideal for addressing many challenges in modern logistics. A blockchain-enabled platform provides:
- Immutable records of shipment data, ensuring that every transaction and movement in the supply chain is recorded accurately and cannot be altered, reducing the risk of fraud and errors
- Enhanced security and transparency, allowing all authorized parties in the supply chain to access real-time, trustworthy information about shipments, reducing disputes and improving collaboration
- Improved traceability of goods, enabling businesses and consumers to track products from their origin to the final destination, which is particularly valuable for industries dealing with sensitive or high-value goods
- Smart contracts that automate and enforce agreement terms, streamlining processes such as payments, customs clearance, and regulatory compliance
- Decentralized data storage that enhances data security and reduces the risk of system-wide failures or data loss
This tool will be particularly useful for businesses dealing with complex international shipping routes. Blockchain technology in supply chain management offers unprecedented levels of transparency and trust, which are crucial in today’s global marketplace. By providing a single source of truth for all supply chain participants, blockchain-enabled platforms can significantly reduce delays, disputes, and inefficiencies in the shipping process. This technology also supports sustainability initiatives by enabling easy verification of product origins and ethical sourcing practices. As regulatory requirements become more stringent and consumers demand greater transparency, blockchain will play a pivotal role in meeting these needs while improving overall supply chain efficiency.
IoT-Driven Shipment Monitoring System
The Internet of Things (IoT) will revolutionize shipment data analysis by providing real-time, detailed data on shipments throughout their journey. This technology enables a level of monitoring and control previously unattainable in logistics. An IoT-driven monitoring system offers:
- Real-time tracking of environmental conditions such as temperature, humidity, and light exposure, which is crucial for sensitive shipments like pharmaceuticals or perishable goods
- Predictive maintenance for shipping containers and vehicles, using sensor data to anticipate equipment failures before they occur and schedule proactive maintenance
- Automated alerts for potential issues, enabling quick responses to anomalies such as unexpected temperature changes, impacts, or deviations from planned routes
- Detailed insights into handling processes, allowing businesses to identify and address inefficiencies in loading, unloading, and transfer operations
- Integration with blockchain technology for enhanced security and traceability of high-value or sensitive shipments
This tool will be crucial for businesses looking to enhance their USPS tracking capabilities and ensure the integrity of sensitive shipments. IoT-driven shipment monitoring systems provide unprecedented visibility into the condition and location of goods throughout the supply chain. This level of insight not only helps in maintaining the quality and safety of shipped products but also enables businesses to optimize their logistics processes, reduce waste, and improve customer satisfaction. As the technology continues to evolve, we can expect to see even more sophisticated applications of IoT in shipping, such as autonomous vehicles and advanced robotics in warehousing and last-mile delivery.
Advanced Returns Management System
An efficient returns process is vital for customer satisfaction and operational efficiency in the e-commerce era. As online shopping continues to grow, the importance of a streamlined, customer-friendly returns process cannot be overstated. An advanced returns management system provides:
- Automated returns authorization, simplifying the process for customers and reducing the workload on customer service teams
- Easy-to-use customer portal for initiating returns, allowing customers to quickly generate return labels and track the status of their returns
- Analytics on return reasons and trends, enabling businesses to identify and address product issues or improve descriptions to reduce return rates
- Integration with inventory management systems for seamless restocking and resale of returned items
- Customizable rules engine for determining return eligibility and processing methods based on factors such as product type, condition, and customer history
This tool will help businesses streamline their TNT tracking and returns process, improving customer satisfaction and reducing operational costs. Advanced returns management systems not only make the returns process more efficient but also provide valuable insights that can help businesses improve their products, reduce return rates, and enhance the overall customer experience. By automating and optimizing the returns process, companies can turn what is often seen as a cost center into an opportunity for customer retention and even additional sales through effective exchange and upsell strategies.
Machine Learning-Based Demand Forecasting Tool
Accurate demand forecasting is crucial for efficient inventory management and overall supply chain optimization. Machine learning algorithms can process vast amounts of data to identify patterns and make predictions with a level of accuracy that far surpasses traditional forecasting methods. A machine learning-based tool offers:
ML-Powered Demand Forecasting Revolution
Traditional Forecasting
Basic statistical methods with limited variables and manual adjustments, leading to higher error rates and outdated predictions.
Machine Learning Advantage
Multi-dimensional analysis incorporating thousands of variables, self-improving algorithms, and real-time adaptation to market changes.
Business Impact
Reduced inventory costs, minimized stockouts, optimized pricing strategies, and improved customer satisfaction through reliable product availability.
Future Evolution
Integration with autonomous supply chains, predictive supplier management, and hyper-personalized inventory allocation based on individual customer forecasts.
Advanced Data Integration Capabilities
- Multi-source Data Harmonization: Sophisticated ETL (Extract, Transform, Load) processes that combine disparate data sources including POS transactions, ERP records, website traffic, social media mentions, and competitor pricing into a unified forecasting dataset.
- External Factor Correlation: Automated identification of correlations between demand patterns and external factors such as weather events, macroeconomic indicators, industry news, and social media trends that traditional forecasting methods typically cannot incorporate.
- Unstructured Data Processing: Natural language processing capabilities that extract relevant demand signals from customer reviews, support tickets, sales team notes, and industry publications to capture qualitative factors affecting product demand.
- Real-time Data Streaming: Continuous integration of live data streams from sales channels, inventory systems, and market sources to enable dynamic forecast updates without waiting for traditional end-of-day or weekly processing cycles.
- Historical Pattern Recognition: Deep learning algorithms that identify complex patterns in historical sales data across multiple timeframes simultaneously, detecting subtle relationships between seemingly unrelated events and subsequent demand fluctuations.
Seasonal Trend Analysis Capabilities
- Multi-dimensional Seasonality Detection: Algorithms that simultaneously identify multiple overlapping seasonal patterns operating at different time scales (daily, weekly, monthly, quarterly, annual) without requiring predefined seasonal periods.
- Event Impact Quantification: Automated measurement of how specific events (holidays, promotions, industry conferences) affected historical demand, with the ability to project these impacts forward while accounting for changing conditions.
- Weather Pattern Integration: Dynamic incorporation of short and long-range weather forecasts combined with historical weather-demand correlations to adjust inventory distribution based on predicted regional weather patterns.
- Cultural Calendar Awareness: Global cultural intelligence that accounts for region-specific holidays, observances, and events that can significantly impact demand patterns in different geographical markets.
- Emerging Trend Identification: Early detection of new seasonal patterns forming in the data before they become obvious, giving businesses a competitive advantage in preparing for emerging cycles in consumer behavior.
Inventory Management Integration
- Automated Reorder Point Optimization: Machine learning algorithms that continuously recalculate optimal reorder points and quantities based on updated demand forecasts, lead time variability, and carrying cost considerations.
- Multi-echelon Inventory Optimization: Sophisticated models that optimize inventory levels across complex supply chain networks, balancing stock levels at distribution centers, regional warehouses, and retail locations to minimize total system costs.
- Safety Stock Dynamic Calculation: Adaptive safety stock algorithms that adjust inventory buffers based on forecast accuracy, supply reliability, and the financial impact of potential stockouts for each product category.
- Inventory Allocation Prioritization: Intelligent allocation systems that distribute limited inventory among competing locations based on forecasted demand, strategic importance, and profitability considerations.
- Slow-moving Inventory Identification: Predictive analytics that flag products trending toward obsolescence before they become excess inventory, enabling proactive markdown or reallocation strategies.
Dynamic Pricing Capabilities
- Demand Elasticity Modeling: Machine learning algorithms that continuously measure how price changes affect demand for each product across different customer segments, time periods, and market conditions.
- Competitive Pricing Intelligence: Automated monitoring of competitor pricing strategies with AI-driven analysis of how those strategies influence the market, enabling proactive rather than reactive pricing adjustments.
- Markdown Optimization: Predictive analytics that determine the optimal timing and depth of price reductions to maximize revenue from seasonal, slow-moving, or end-of-life inventory based on remaining forecasted demand.
- Bundle Pricing Optimization: Advanced algorithms that identify high-value product combinations and calculate optimal bundle pricing to increase average order value while clearing complementary inventory at appropriate rates.
- Channel-specific Pricing Strategies: Differentiated pricing models for each sales channel based on channel-specific demand patterns, competitive landscapes, and customer price sensitivity profiles.
Scenario Modeling Capabilities
- What-If Analysis Tools: Interactive simulation capabilities that allow supply chain planners to model the impact of potential disruptions, market changes, or business decisions on demand patterns and inventory requirements.
- Market Shock Simulation: Stress-testing functionality that models how extreme events (natural disasters, major economic shifts, competitor actions) would affect demand and supply chain performance based on historical response patterns.
- New Product Launch Modeling: Predictive algorithms that project demand curves for new products based on similarities to existing products, market research, and early sales indicators, reducing new product forecasting uncertainty.
- Cannibalization and Halo Effect Quantification: Sophisticated analysis of how new products or promotions affect demand for existing products, capturing both negative (cannibalization) and positive (halo) effects across the product portfolio.
- Supply Chain Reconfiguration Testing: Virtual supply chain environment for testing how changes to sourcing strategies, manufacturing locations, or distribution networks would affect overall supply chain responsiveness to forecasted demand.
This tool will be essential for businesses looking to optimize their Expeditors tracking and inventory management. By leveraging machine learning for demand forecasting, businesses can significantly improve their inventory management, reduce carrying costs, and enhance their ability to meet customer demand. This technology enables companies to move from reactive to proactive supply chain management, anticipating changes in demand and adjusting their operations accordingly. As these systems become more sophisticated, they will play an increasingly critical role in supply chain optimization, helping businesses navigate the complexities of global markets and rapidly changing consumer preferences.
Post-Purchase Analytics Platform
Understanding customer behavior after purchase is crucial for improving retention and driving long-term business growth. A post-purchase analytics platform provides deep insights into the customer journey beyond the point of sale, enabling businesses to optimize their post-purchase experience and build stronger customer relationships. This platform provides:
Post-Purchase Journey Analytics
Order Confirmation
Analysis of email open rates, click-through behavior, and upsell acceptance during the initial confirmation phase.
Tracking Engagement
Measurement of tracking page visits, time spent reviewing status, and interaction with supplementary content.
Delivery Experience
Tracking of delivery confirmation interactions, unboxing behavior, and initial product engagement patterns.
Post-Delivery Engagement
Measurement of review submission, support interactions, social sharing, and repeat purchase behavior.
Post-Purchase Behavior Analysis
- Tracking Page Interaction Mapping: Detailed analysis of how customers engage with order tracking interfaces, including visit frequency, time spent per visit, interaction patterns, and correlation with support inquiries or satisfaction scores.
- Delivery Experience Assessment: Measurement of customer responses to different delivery scenarios, quantifying the impact of early deliveries, delays, or specific carrier experiences on subsequent customer behavior and sentiment.
- Unboxing and Initial Usage Tracking: Analysis of product activation rates, initial usage patterns, and feature discovery sequences to identify potential friction points in the initial product experience that may affect long-term satisfaction.
- Return Behavior Prediction: Advanced algorithms that identify return probability based on post-purchase engagement patterns, product category performance, and customer history, enabling proactive interventions before return initiation.
- Voice of Customer Integration: Holistic combination of explicit feedback (reviews, surveys, support interactions) with implicit behavioral signals to create a comprehensive understanding of post-purchase satisfaction drivers.
Cross-Selling and Upselling Opportunity Identification
- Purchase Sequence Analysis: Machine learning algorithms that identify common product purchase sequences across customer segments, revealing natural progression patterns that can inform targeted cross-sell recommendations.
- Usage-Based Recommendation Engine: Systems that analyze actual product usage data (when available) to suggest relevant complementary products based on demonstrated use patterns rather than just purchase history.
- Timing Optimization Models: Predictive analytics that determine the ideal timing for different types of cross-sell or upsell offers based on purchase cycle analysis, product category relationships, and individual customer engagement patterns.
- Bundle Effectiveness Measurement: Comprehensive tracking of how different product bundles and complementary offers perform across various customer segments and purchase contexts to continuously refine bundling strategies.
- Cross-Category Opportunity Detection: Advanced pattern recognition that identifies non-obvious relationships between purchases in seemingly unrelated categories, uncovering novel cross-selling opportunities that basic recommendation engines would miss.
Post-Purchase Communication Impact Measurement
- Multi-touch Attribution Modeling: Sophisticated analysis that accurately attributes the influence of various post-purchase communications (order confirmations, shipping updates, follow-up emails) on key metrics like repeat purchases, reviews, and referrals.
- Content Effectiveness Analysis: Detailed measurement of how different content types, formats, and messaging approaches in post-purchase communications affect engagement rates, sentiment, and subsequent customer behaviors.
- Optimal Frequency Determination: Machine learning algorithms that identify the ideal communication cadence for different customer segments and purchase types, balancing information needs with potential messaging fatigue.
- Cross-Channel Consistency Tracking: Evaluation of messaging consistency and customer experience across email, SMS, app notifications, and web interfaces throughout the post-purchase journey to identify potential disconnects.
- A/B Testing Infrastructure: Comprehensive experimentation framework for continuously optimizing post-purchase communications through controlled testing of variables like timing, content, personalization level, and call-to-action approaches.
Customer Segmentation Capabilities
- Behavioral Cohort Analysis: Advanced segmentation that groups customers based on similar post-purchase behavior patterns rather than traditional demographic or purchase value segments, revealing actionable behavioral insights.
- Satisfaction-Based Segmentation: Classification of customers based on their expressed and implied satisfaction levels throughout the post-purchase journey, enabling targeted retention strategies for at-risk segments.
- Engagement Depth Profiling: Multi-dimensional analysis of how deeply customers engage with different aspects of the post-purchase experience, from superficial tracking to deep product education and community participation.
- Channel Preference Identification: Automated detection of individual customer preferences for post-purchase communication channels and interaction touchpoints, enabling personalized engagement strategies.
- Dynamic Segment Evolution Tracking: Continuous monitoring of how customer segments evolve over time and in response to business changes, product launches, or market conditions, ensuring segmentation strategies remain relevant.
Predictive Analytics for Customer Lifetime Value
- Early Indicator Identification: Machine learning models that identify subtle behavioral signals in the first post-purchase interactions that correlate strongly with long-term customer value and retention probability.
- Retention Risk Scoring: Real-time calculation of churn risk based on post-purchase engagement patterns, product category performance, and comparative analysis with similar customer cohorts.
- Next Purchase Prediction: Advanced algorithms that forecast when a customer is likely to make their next purchase and in which category, enabling perfectly timed remarketing and retention campaigns.
- Lifetime Value Trajectory Modeling: Sophisticated projection of how customer value is likely to evolve over time based on initial post-purchase signals, comparable customer journeys, and identified growth patterns.
- Intervention Impact Forecasting: Predictive models that estimate the potential return on investment for different types of retention interventions at various points in the customer journey, optimizing resource allocation.
Implementation and Integration Considerations
- Cross-Platform Data Unification: Comprehensive data integration capabilities that combine signals from e-commerce platforms, shipping carriers, customer service systems, and marketing automation tools into a unified post-purchase view.
- Privacy-Centric Design: Built-in compliance with global privacy regulations including anonymization options, consent management, and transparent data usage policies that maintain customer trust while enabling powerful analytics.
- Action-Oriented Dashboards: Intuitive visualization interfaces designed for different business stakeholders, highlighting actionable insights and direct paths to implementing recommended interventions rather than just displaying metrics.
- Business System Integration: Seamless connections with existing business systems including CRM platforms, marketing automation tools, and customer service software to enable coordinated action based on analytics insights.
- Continuous Learning Framework: Self-improving analytics models that continuously refine their predictions and recommendations based on observed outcomes, ensuring the platform becomes more valuable over time.
This tool will help businesses leverage their post-purchase data for improved customer retention. By focusing on the post-purchase experience, companies can increase customer satisfaction, encourage repeat purchases, and build brand loyalty. The insights provided by post-purchase analytics platforms enable businesses to create more personalized and engaging customer experiences, turning one-time buyers into loyal, long-term customers. As competition in the e-commerce space intensifies, the ability to effectively manage and optimize the post-purchase experience will become a key differentiator for successful businesses.
Sustainability-Focused Shipping Analytics Tool
Improved Efficiency
Real-time tracking and AI-powered analytics optimize routes and streamline operations
Cost Reduction
Carrier performance analytics and comparative analysis help identify cost-effective options
Enhanced Customer Experience
Real-time updates and predictive delivery estimates improve customer satisfaction
Increased Security
Blockchain technology enhances traceability and security for complex shipments
As sustainability becomes increasingly important to both consumers and businesses, a dedicated analytics tool for eco-friendly shipping will be crucial. This tool helps companies balance their operational efficiency with environmental responsibility, providing insights and recommendations to reduce the environmental impact of shipping operations. This tool offers:
- Carbon footprint tracking for shipments, allowing businesses to measure and report on the environmental impact of their logistics operations
- Analysis of eco-friendly packaging utilization, helping companies optimize their packaging choices to reduce waste and improve sustainability
- Recommendations for reducing environmental impact, such as suggesting alternative routes or transportation methods that reduce emissions
- Integration with carrier selection tools to prioritize carriers with strong sustainability practices
- Reporting capabilities for sustainability metrics, supporting corporate social responsibility initiatives and regulatory compliance
This tool will help businesses align their shipping practices with sustainability goals and enhance their brand image. As consumers become increasingly environmentally conscious, businesses that can demonstrate a commitment to sustainable shipping practices will have a competitive advantage. Sustainability-focused shipping analytics tools not only help companies reduce their environmental impact but also often lead to cost savings through more efficient resource utilization. By providing clear insights into the environmental implications of shipping decisions, these tools enable businesses to make informed choices that balance economic and ecological considerations.
Comparison Table of Shipment Data Analysis Tools
Tool | Key Features | Best For |
---|---|---|
WISMOlabs | All-in-one solution, real-time tracking, returns management | Comprehensive shipment data analysis |
AI-Powered Predictive Analytics | Demand forecasting, route optimization | Forward-looking shipping strategies |
Real-Time Tracking Solution | Instant updates, predictive delivery estimates | Enhancing customer experience |
Carrier Performance Analytics | Comparative analysis, cost-efficiency metrics | Optimizing carrier selection |
Blockchain-Enabled Platform | Enhanced security, improved traceability | Complex international shipping |
Frequently Asked Questions
1. How do these tools integrate with existing e-commerce platforms?
Most of these tools offer APIs and custom connectors for easy integration with major e-commerce platforms. They typically work with popular platforms like Shopify, WooCommerce, and Magento, allowing businesses to easily add advanced shipment data analysis to their current systems. These integrations often support real-time data syncing, keeping information up-to-date across all systems.
2. Can these tools handle international shipping data?
Yes, many of these tools, especially the blockchain-enabled and IoT-driven systems, are designed to handle complex international shipping routes and provide global visibility. They often include features specifically for international logistics, such as multi-currency support, compliance with international shipping rules, and the ability to handle different data formats from various countries and carriers. These tools can typically manage the complexities of cross-border shipping, including customs documentation, duty calculations, and international tracking across multiple carriers.
3. How do these tools help in reducing shipping costs?
By providing detailed carrier performance analytics, route optimization, and demand forecasting, these tools help businesses find the most cost-effective shipping options and improve overall efficiency. They often include features like rate comparison across multiple carriers, historical cost analysis, and predictive modeling to anticipate future shipping costs. Additionally, by optimizing routes and improving inventory management based on accurate demand forecasts, these tools can help reduce unnecessary transportation and storage costs.
4. Are these tools suitable for small businesses?
Yes, many of these tools offer scalable solutions that can be adapted to meet the needs of businesses of all sizes, from small startups to large enterprises. Vendors often provide tiered pricing models and customizable feature sets, allowing small businesses to access advanced analytics capabilities without overwhelming costs or complexity. Some tools also offer specific packages designed for small and medium-sized enterprises, focusing on essential features that provide the most value for smaller operations.
5. How do these tools contribute to improving customer satisfaction?
These tools enhance customer satisfaction through features like real-time tracking, proactive notifications, easy returns management, and improved delivery accuracy. By giving customers timely and accurate information about their shipments, businesses can reduce anxiety and increase trust. Advanced analytics also enable businesses to spot and address potential issues before they affect the customer, leading to smoother shipping experiences and fewer customer service inquiries. Additionally, features like easy-to-use return portals and personalized post-purchase communications contribute to a positive overall customer experience.
Conclusion: Transforming Logistics Through Advanced Data Analytics
As we approach 2025, the evolution of shipment data analysis tools represents a fundamental shift in how businesses approach logistics management. These sophisticated platforms are no longer mere operational tools but strategic assets that drive competitive advantage in an increasingly complex global marketplace. By harnessing the power of artificial intelligence, machine learning, IoT connectivity, and blockchain verification, forward-thinking companies can transform raw shipping data into actionable intelligence that optimizes operations, enhances customer experiences, and drives sustainable growth.
The Strategic Impact of Advanced Shipment Analytics
- Transforms reactive logistics operations into proactive, data-driven decision frameworks
- Elevates shipping from a cost center to a strategic differentiator that drives customer loyalty
- Creates predictive capabilities that mitigate disruptions before they affect customers
- Enables precision inventory management that balances working capital against service levels
- Provides the foundation for sustainable logistics practices with measurable environmental impacts
- Bridges organizational silos by connecting shipping data with marketing, sales, and customer service intelligence
The convergence of these technologies is creating unprecedented visibility throughout the supply chain, enabling businesses to make informed decisions in real-time that optimize every aspect of their shipping operations. From demand forecasting and route optimization to carrier selection and post-purchase engagement, these tools provide the comprehensive insights needed to navigate the complexities of modern logistics with confidence and precision. As customer expectations continue to rise and supply chains become increasingly global and interconnected, the ability to effectively analyze and act on shipment data will be a critical determinant of business success.
Whether you’re looking to enhance your DHL tracking capabilities, streamline FedEx operations, optimize carrier performance, or implement a comprehensive shipment analytics strategy, the tools outlined in this article provide the foundation for logistics excellence in the digital age. By embracing these innovative solutions, businesses of all sizes can position themselves to thrive in an environment where shipping efficiency, transparency, and sustainability are increasingly critical to customer satisfaction and business success.
The future of logistics belongs to organizations that can effectively harness the power of data to create shipping experiences that are not just efficient but exceptional. As these advanced analytics tools continue to evolve and become more accessible, they will democratize access to sophisticated logistics capabilities, enabling businesses of all sizes to compete effectively in the global marketplace. By investing in the right shipment data analysis tools today, companies can build the foundation for logistics excellence that will drive competitive advantage well into the future.